Leveraging Network Detection and Deep Learning for Insurance Fraud Prevention
Insurance fraud evolves, blending with legitimate claims. Sophisticated tech solutions crucial. Hudson Data's ML-Graph & Model Foundry lead in detection and prevention.
Introduction
Insurance fraud has evolved into a complex challenge with fraudsters often mixing fraudulent claims with legitimate ones, making detection particularly difficult. This dynamic requires sophisticated technological solutions. By integrating predictive analytics with advanced technologies like network detection and deep learning, we can enhance the ability to detect and prevent fraud. Hudson Data's ML-Graph and Model Foundry platforms are at the forefront of this battle, providing innovative solutions to these intricate problems.
The Complexity of Insurance Fraud
Insurance fraud significantly impacts the industry both financially and in terms of consumer trust. A typical scenario involves providers who submit both fraudulent and legitimate claims to camouflage their deceitful activities. This mixture complicates the detection process, as outright blocking of all claims from such providers would also stop legitimate claims, harming innocent parties and disrupting business operations.
Hudson Data’s ML-Graph: Network Detection Explained
ML-Graph, developed by Hudson Data, uses network analysis to detect unusual patterns and relationships that indicate fraudulent networks. This tool is particularly adept at unraveling the complex web of transactions and identifying inconsistencies in data that suggest fraudulent activities. By analyzing the vast networks of claims and their connections, ML-Graph helps insurers pinpoint potential fraud within massive datasets, providing actionable insights even when legitimate and fraudulent claims are intermixed.
Model Foundry: Deep Learning for Outlier Detection
Complementing ML-Graph, Hudson Data's Model Foundry employs deep learning techniques to identify outliers in data—claims that deviate from the norm which may suggest fraudulent activity. These models are trained on large datasets to recognize subtle patterns that differentiate legitimate from fraudulent claims. Through continual learning and adaptation, these models become increasingly effective at detecting anomalies, significantly enhancing the accuracy of fraud detection in complex claim scenarios.
Integrating ML-Graph and Model Foundry
Integrating ML-Graph with Model Foundry creates a robust framework for detecting fraud. ML-Graph's network detection capabilities identify suspicious networks of transactions, while Model Foundry's deep learning models analyze individual claims within these networks to detect outliers. This dual approach allows for nuanced understanding and processing of claims, ensuring accurate detection without disrupting legitimate claims. This integration not only increases detection rates but also speeds up the processing time, making real-time fraud detection feasible.
Operational Challenges and Solutions
Implementing these technologies presents several challenges, including integration with existing systems, managing data privacy, and ensuring the accuracy of the models. Solutions include using advanced encryption for data security, regular updates to the models to adapt to new fraudulent tactics, and training for staff to handle these sophisticated systems. Overcoming these challenges is crucial for the successful deployment of AI and ML in fighting insurance fraud.
Future Directions and Innovations
The future of fraud detection in insurance looks promising with advancements in AI technologies like explainable AI, which provides transparency in AI decisions, and federated learning, which enhances privacy in collaborative environments. These technologies will further refine fraud detection processes, making them more accurate and user-friendly.
Conclusion
The integration of Hudson Data’s ML-Graph and Model Foundry platforms represents a significant advancement in the fight against insurance fraud. By leveraging network detection and deep learning, insurers can effectively tackle the challenges posed by mixed fraudulent and legitimate claims. Continued investment and innovation in these technologies are imperative for enhancing security and operational efficiency, ultimately fostering a safer insurance landscape for all stakeholders.
This article serves as a comprehensive guide to understanding and implementing advanced AI-driven solutions for the complex challenges of insurance fraud detection.
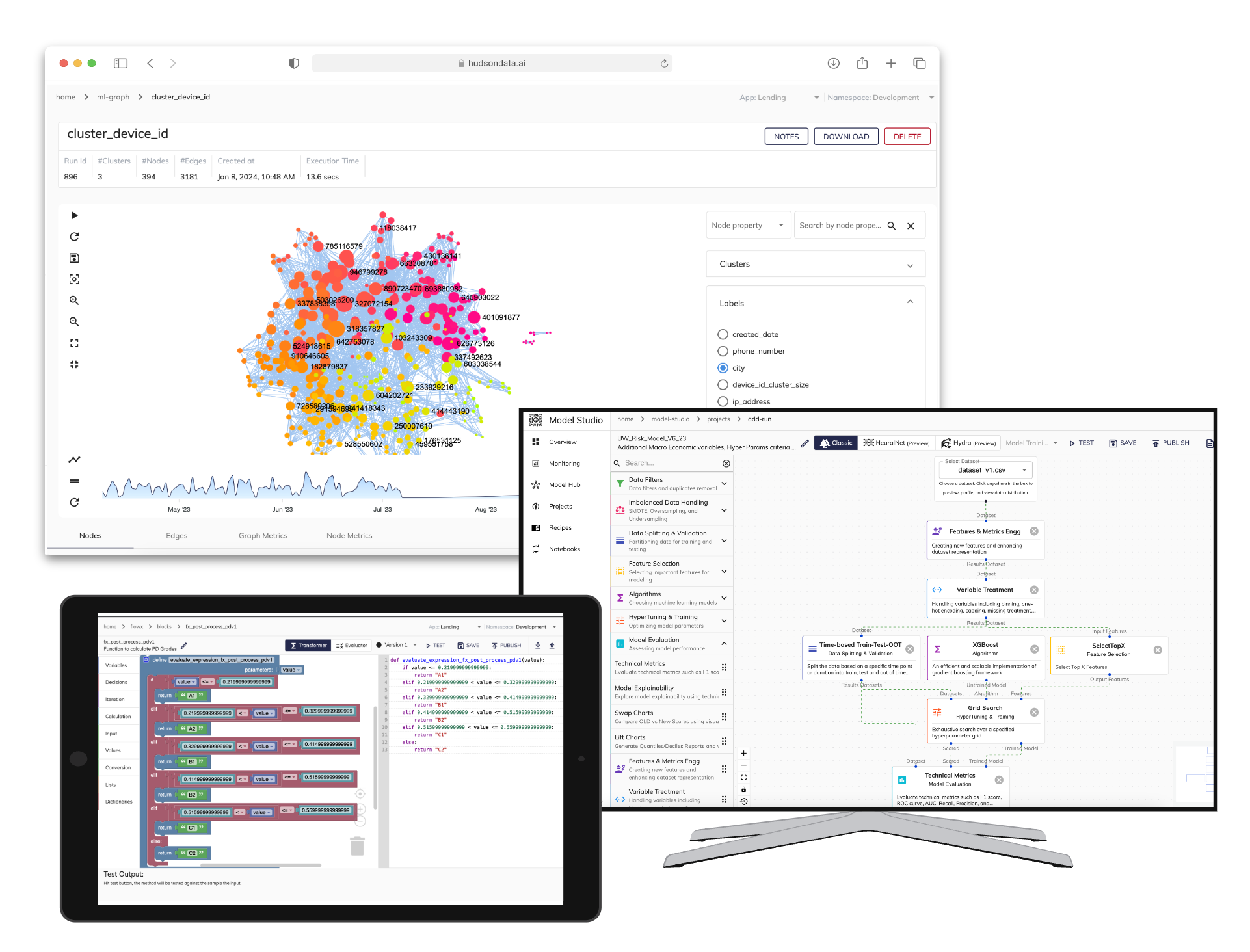